Today I am at the Health Data Analytics summit. The title of the keynote talk is Achieving Care transformation by Infusing Electronic Health Records with Wisdom. It’s a delight to hear from a medical informaticist: David M. Liebovitz (publications in Google Scholar), MD, FACP, Chief Medical Information Officer, The University of Chicago. He graduated from University of Illinois in electrical engineering, making this a timely talk as the engineering-focused Carle Illinois College of Medicine gets going.
David Liebovitz started with a discussion of the data problems — problem lists, medication lists, family history, rules, results, notes — which will be familiar to anyone using EHRs or working with EHR data. He draws attention also to the human problems — both in terms of provider “readiness” (e.g. their vision for population-level health) as well as about “current expectations”. (An example of such an expectation is a “main clinician satisfier” he closed with: U Chicago is about to turn on outbound faxing from the EHR!) He mentioned also the importance of resilience.
He mentioned customizing systems as a risk when the vendor makes upstream changes (this is not unique to healthcare but a threat to innovation and experimentation with information systems in other industries.) Still, in managing the EHR, there is continual optimization, scored based on a number of factors. He mentioned:
- Safety
- Quality/patient experience
- Regulatory/legal
- Financial
- Usability/productivity
- Availability of alternative solutions
As well as weighting for old requests.
He emphasized the complexity of healthcare in several ways:
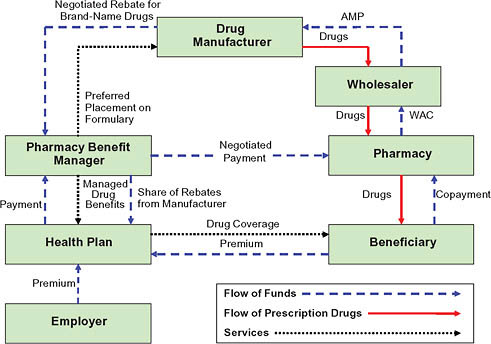
An image from “Prices That Are Too High”, Chapter 5, The Healthcare Imperative: Lowering Costs and Improving Outcomes: Workshop Series Summary (2010)
- Icosystem’s diagram of the complexity of the healthcare system

Icosystem – complexity of the healthcare system
- Another complexity is the modest impact of medical care compared to other factors
- such as the impact of socioeconomic and political context on equity in health and well-being (see the WHO image below).
- For instance, there is a large impact of health behaviors, which “happen in larger social contexts.” (See the Relative Contribution of Multiple Determinants to Health, August 21, 2014, Health Policy Briefs)
Given this complexity, David Liebovitz stresses that we need to start with the right model, “simultaneously improving population health, improving the patient experience of care, and reducing per capita cost”. (See Stiefel M, Nolan K. A Guide to Measuring the Triple Aim: Population Health, Experience of Care, and Per Capita Cost. IHI Innovation Series white paper. Cambridge, Massachusetts: Institute for Healthcare Improvement; 2012).
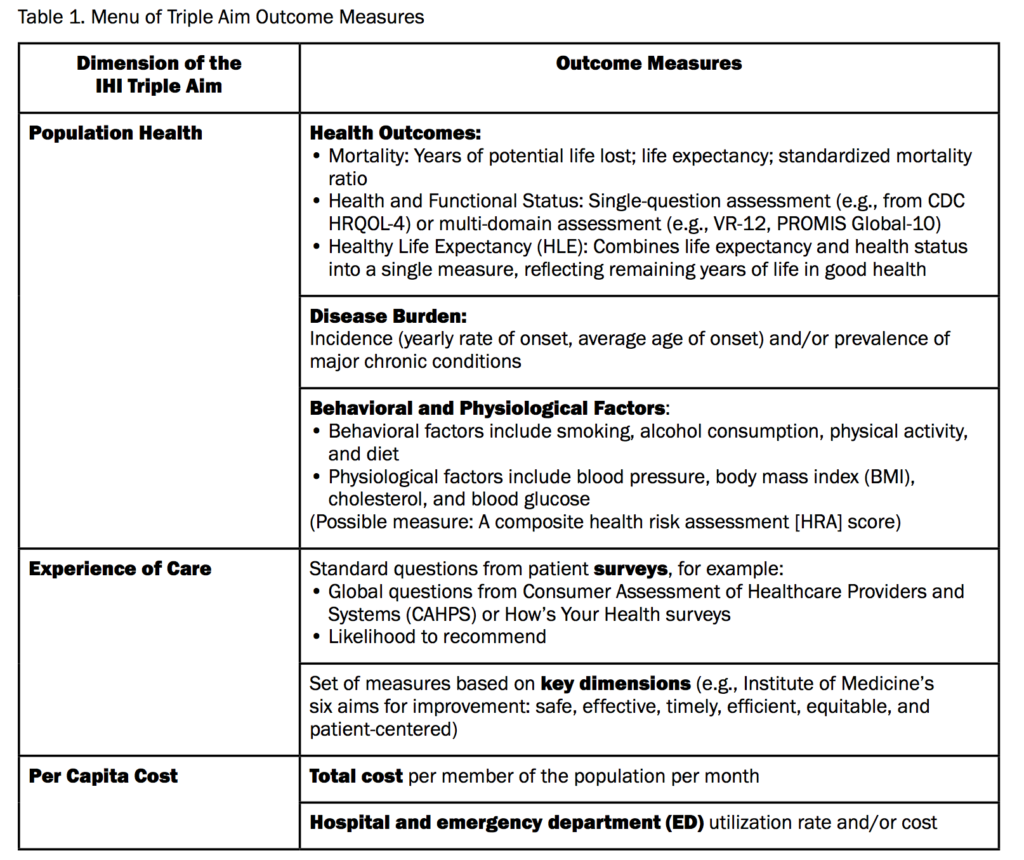
Table 1 from Stiefel M, Nolan K. A Guide to Measuring the Triple Aim: Population Health, Experience of Care, and Per Capita Cost. IHI Innovation Series white paper. Cambridge, Massachusetts: Institute for Healthcare Improvement; 2012.
Given the modest impact of medical care, and of data, he suggests that we should choose the right outcomes.
David Liebovitz says that “not enough attention has been paid to usability”; I completely agree and suggest that information scientists, human factors engineeers, and cognitive ergonomists help mainstream medical informaticists fill this gap. He put up Jakob Nielsen’s 10 usability heuristics for user interface design A vivid example is whether a patient’s resuscitation preferences are shown (which seems to depend on the particular EHR screen): the system doesn’t highlight where we are in the system. For providers, he says user control and freedom are very important. He suggests that there are only a few key tasks. A provider should be able to do ANY of these things wherever they are in the chart:
- put a note
- order something
- send a message
Similarly, EHR should support recognition (“how do I admit a patient again?”) rather than requiring recall.
Meanwhile, on the decision support side he highlights the (well-known) problems around interruptions by saying that speed is everything and changing direction is much easier than stopping. Here he draws on some of his own work, describing what he calls a “diagnostic process aware workflow”
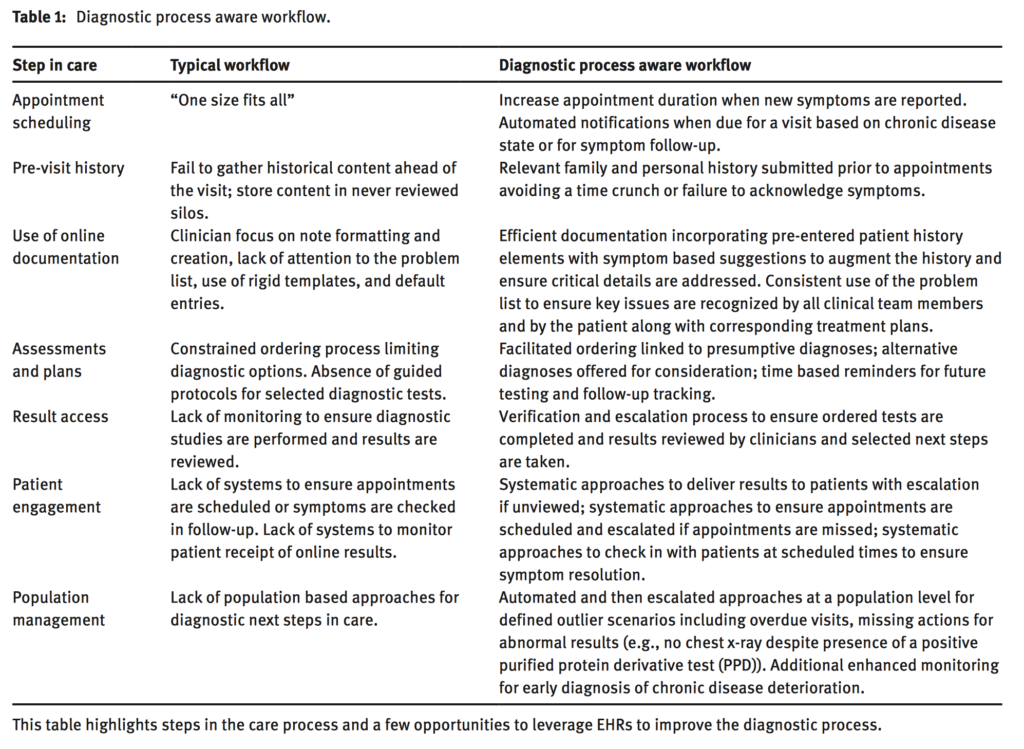
David Liebovitz. Next steps for electronic health records to improve the diagnostic process. Diagnosis 2015 2(2) 111-116. doi:10.1515/dx-2014-0070
Can we predict X better? Yes, he says (for instance pointing to Table 3 of “Can machine-learning improve cardiovascular risk prediction using routine clinical data?” and its machine learning analysis of over 300,000 patients, based on variables chosen from previous guidelines and expert-informed selection–generating further support for aspects such as aloneness, access to resources, socio-economic status). But what’s really needed, he says, is to:
- Predict the best next medical step, iteratively
- Predict the best next lifestyle step, iteratively
- (And what to do about genes and epigenetic measures?)
He shows an image of “All of our planes in the air” from flightaware, drawing the analogy that we want to work on “optimal patient trajectories” — predicting what are the “turbulent events” to avoid”. This is not without challenges. He points to three:
He closes suggesting that we:
- Finish the basics
- Address key slices of the spectrum
- Descriptive/prescriptive
- Begin the prescriptive journey: impact one trajectory at a time.